Economic Implications of Algorithmic Trading
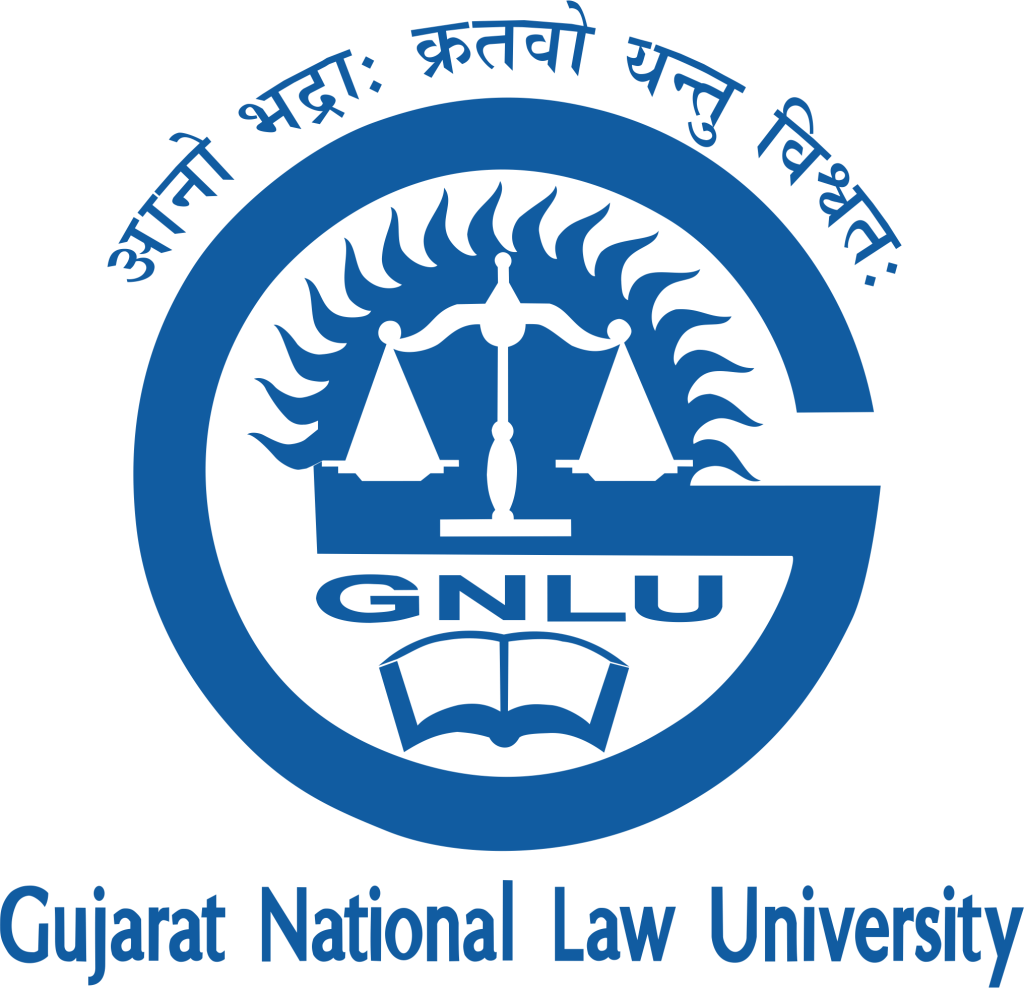
-Arihant Sethia and Pankti Rupani
Introduction
Algorithmic trading has become an integral part of the financial industry, revolutionizing the way trades are executed and impacting various aspects of the economy. In this article, we will explore the economic implications of algorithmic trading, examining its growth, mechanics, benefits, challenges, and its impact on financial markets and institutions.
Algorithmic trading traces its origins back to the 1970s when computer technology started playing a significant role in financial markets. Initially, it was mainly used by institutional investors and large financial firms to automate trading processes and improve efficiency. Over the years, algorithmic trading has grown exponentially, thanks to technological advancements and the availability of high-speed computing. With the integration of sophisticated algorithms and increased data processing capabilities, algorithmic trading has become more accessible to a wider range of market participants.
Algorithmic trading systems consist of various components, including market data feeds, execution algorithms, and order management systems. Market data feeds provide real-time pricing information, while execution algorithms determine the optimal execution strategy for trades. Order management systems handle the submission and tracking of orders.
Algo Trading has a substantial impact on modern financial markets. They enhance price efficiency by capitalizing on permanent price shifts and rectifying transient pricing errors through marketable orders. However, their liquidity-supplying nonmarketable orders are adversely selected. HFTs’ informational advantage empowers them to generate positive trading revenues, surpassing bid-ask spreads and fees. Nonetheless, concerns persist about whether the informational gains outweigh the adverse selection costs imposed on non-HFT participants. They often help reduce pricing errors, although adverse selection costs may drive non-HFT liquidity suppliers away. This indirect consequence could impact market stability.
Algorithmic trading relies on a range of algorithms and strategies to execute trades. These include trend-following algorithms, mean-reversion algorithms, and statistical arbitrage strategies. Each algorithm is designed to exploit different market conditions and generate profits. Data analysis and prediction models play a crucial role in algorithmic trading. By analysing vast amounts of historical and real-time data, traders can identify patterns and trends, enabling them to make more informed trading decisions. Prediction models help forecast market movements and guide algorithmic strategies.
Benefits of Algorithmic Trading
Algorithmic trading has significantly improved market liquidity and efficiency. By executing trades at high speeds, algorithmic traders provide liquidity to markets, ensuring smoother transactions and tighter bid-ask spreads. This increased liquidity benefits all market participants.
With the automation of trades, algorithmic trading has led to a reduction in trading costs. Algorithmic trading has revolutionized the financial landscape, offering an efficient means of executing trading strategies. However, a crucial aspect that often gets overlooked is the consideration of transaction costs. In the realm of algorithmic trading, these costs encompass both explicit and implicit components.
Explicit transaction costs are the more apparent ones, such as commissions, brokerage fees, taxes, and clearing and settlement fees. While these are relatively straightforward to incorporate into a back testing engine, they can have a substantial impact on a strategy’s profitability. Ignoring or underestimating these costs can lead to a significant gap between simulated and real-world results.
Implicit transaction costs are often the silent killers for algorithmic trading strategies. These costs include slippage, latency, and market impact. Slippage represents the difference between the intended trade price and the actual executed price due to delays in order execution. Latency is the time lag between a trade decision and its execution, which can be especially critical for high-frequency strategies. Market impact, on the other hand, is the cost incurred when a trade impacts the supply and demand dynamics of the market, influencing prices. These costs can be a make-or-break factor for the success of a strategy.
Therefore, transaction costs are a critical consideration in algorithmic trading, and their proper incorporation is essential for accurate back testing and real-world trading success. By addressing both explicit and implicit costs, algorithmic traders can work towards optimizing their strategies and achieving better results in live markets.
Challenges and Risks in Algorithmic Trading
One of the critical challenges in algorithmic trading is the vulnerability to market crashes and flash crashes. As trading algorithms react to market conditions, sudden swings in prices and liquidity shortages can trigger massive sell-offs, exacerbating market downturns.
Algorithmic trading also poses potential systemic risks and the risk of market manipulation. Complex algorithms interacting in high-speed environments may create unforeseen correlations or amplify existing risks, potentially destabilizing financial markets. Additionally, there is a concern that algorithmic trading can be exploited for market manipulation purposes.
The rise of algorithmic trading has raised regulatory concerns and legal implications. Regulators strive to ensure fair and orderly markets while monitoring the impact of algorithmic trading on market dynamics and integrity. In response, regulations have been implemented to establish safeguards, promote transparency, and mitigate potential risks.
Impact of Algorithmic Trading on Financial Markets
Algorithmic trading has significantly influenced market structure and dynamics. It has increased competition and reduced barriers to entry, allowing smaller firms to compete with larger players. Market fragmentation has also increased as trading avenues have multiplied to accommodate different algorithms and strategies.
The widespread adoption of algorithmic trading has led to significant changes in trading patterns and the behaviour of market participants. Traditional buy-and-hold strategies have been replaced by high-frequency trading, with market participants increasingly relying on algorithms to execute their trades.
Algorithmic trading has had profound effects on market volatility and price fluctuations. While it has contributed to increased liquidity and reduced bid-ask spreads, the automation and speed of algorithmic trading can also amplify short-term price movements and lead to increased volatility, particularly during periods of market stress.
Algorithmic Trading and Market Efficiency
Algorithmic trading challenges the efficient market hypothesis, which assumes that all relevant information is incorporated into market prices at all times. With the ability to quickly process and act on information, algorithms may exploit temporary price inefficiencies, suggesting that markets may not always be perfectly efficient.
Algorithmic trading has had a significant impact on price discovery and market transparency. By processing vast amounts of data and analysing market conditions, algorithms can identify pricing discrepancies and contribute to the discovery of fairer market prices. Furthermore, algorithmic trading has increased market transparency as orders are executed electronically and displayed in real-time.
The rise of algorithmic trading has raised concerns regarding information asymmetry and fair access to markets. Algorithmic traders, with their superior technology and access to data, may have an advantage over traditional traders. Regulators aim to ensure fair access to markets and level the playing field for all participants.
Algorithmic Trading and the Role of Human Traders
Algorithmic trading and traditional trading are not mutually exclusive. Many traders, including financial institutions, have embraced algorithmic trading as a complementary strategy alongside traditional trading approaches. Decisions made by human traders are often based on fundamental analysis and market sentiment, enabling them to add value in specific situations.
The rise of algorithmic trading has led to a shift in job roles and skill requirements within the financial industry. Traders and industry professionals now require a solid understanding of algorithms, data analysis, and programming languages to effectively engage in algorithmic trading activities.
There is a coexistence and collaboration between algorithms and human traders. Algorithms perform tasks efficiently, such as trade execution and risk management, while human traders bring their expertise in fundamental analysis and decision-making. Combining the strengths of both can enhance trading strategies and improve overall performance.
Algorithmic Trading and Financial Institutions
Banks and financial institutions have widely adopted algorithmic trading as part of their investment strategies. These institutions utilize algorithms to execute large trades, achieve better pricing, and manage risks efficiently. Algorithmic trading has become an essential tool for diversifying portfolios and maximizing returns.
Algorithmic trading has significant implications for investment strategies and portfolio management. Sophisticated algorithms enable financial institutions to implement complex trading strategies, diversify portfolios, and optimize investment decisions. The use of algorithms allows for more precise risk analysis and efficient allocation of capital.
Implementing algorithmic trading poses challenges for financial institutions. They must ensure the robustness of algorithms, maintain compliance with regulations, and manage the risks associated with automated trading. Developing and continuously updating algorithms to adapt to changing market conditions is an ongoing challenge for financial institutions.
Algorithmic Trading in Different Financial Markets
Algorithmic trading is extensively used in equities and stock markets. High-frequency trading strategies dominate these markets, where algorithms execute trades based on real-time market data and execute large volumes of trades within fractions of a second.
Algorithmic trading has gained significant traction in foreign exchange and currency trading. The forex market’s high liquidity and 24-hour trading make it an ideal environment for algorithmic traders to execute trades efficiently and capture opportunities across various currency pairs.
Algorithmic trading is also becoming more prevalent in commodity markets and derivatives trading. Algorithms can analyse vast amounts of data, including supply and demand factors, geopolitical events, and price trends, to make informed trading decisions in these highly complex and volatile markets.
Algorithmic Trading and Market Manipulation
Algorithmic trading introduces risks and challenges related to market manipulation. The speed, volume, and complexity of algorithmic transactions can be exploited to manipulate prices, create false market signals, or engage in illegal activities that distort market conditions.
Efforts are being made to detect and prevent algorithmic trading abuses. Market surveillance systems and regulatory measures are continuously being developed to identify suspicious trading patterns, monitor market manipulation, and impose penalties on those found guilty of illegal activities.
Regulators have implemented various regulatory measures and surveillance systems to ensure the integrity of the financial markets. These measures aim to increase transparency, monitor trading activities, and detect any potential market manipulation. The goal is to create an environment that ensures fair and orderly markets for all participants.
Ethics and Social Impact of Algorithmic Trading
Algorithmic trading practices raise ethical considerations. The use of algorithms and high-frequency trading techniques may create a perception of unfair advantages or lead to market manipulation. The industry must address these concerns and adopt ethical frameworks that prioritize fairness, transparency, and accountability.
The rise of algorithmic trading has raised concerns about social implications and inequalities. The increasing dominance of algorithms may reduce employment opportunities for traditional traders and widen the socioeconomic gap. It is crucial to consider the broader societal impact and ensure that algorithmic trading is implemented responsibly.
To mitigate ethical concerns, transparency and accountability should be integral components of algorithmic trading algorithms. Regulatory bodies, financial institutions, and algorithmic traders themselves should ensure that algorithms are auditable, understandable, and comply with ethical standards. Transparency fosters trust and helps address social concerns.
Algorithmic Trading and Financial Crises
Algorithmic trading plays a role in financial crises. During times of market stress, algorithmic trading can contribute to increased volatility and market downturns. The rapid execution of sell orders triggered by algorithms can escalate market instabilities.
Algorithmic trading can exacerbate market downturns and increase systemic risks during financial crises. The interconnectivity of algorithms can lead to cascading effects, amplifying panic selling and intensifying market downturns.
Financial crises have highlighted the need for safeguards and risk management practices in algorithmic trading. Improved regulation, circuit breakers, and robust risk controls are among the measures implemented to address the potential negative impact of algorithmic trading during market downturns.
Future Trends and Developments in Algorithmic Trading
The future of algorithmic trading lies in advancements in artificial intelligence and machine learning. These technologies enable algorithms to evolve and adapt in response to changing market conditions, increasing their predictive capabilities and potential profitability.
The integration of big data and predictive analytics will further enhance algorithmic trading strategies. With access to vast amounts of data, algorithms can make more accurate predictions and improve trading decisions, enabling traders to take advantage of emerging market opportunities.
Quantum computing holds the potential to revolutionize algorithmic trading. The immense computational power of quantum computers can significantly enhance the speed and complexity of trading algorithms, opening up new avenues for profitable trading strate.
The authors are students of Gujarat National Law University, Gandhinagar.